An Upper Left Origin
A native of Oregon, Noah Fahlgren grew up with easy access to the natural world.
Living in the Willamette Valley, he was in the center of an ecologically diverse state: drive an hour west and he was at the coast. An hour east and he was surrounded by mountains. An inherent appreciation of nature and a fascination with science and computers helped to lead him to where he is today, principal investigator and director of our Data Science core facility at the Danforth Center.
From Lab Bench to Data Analysis
Like many scientists, invested teachers became powerful mentors in Noah’s life, and helped define his career. As an undergraduate student, he started working in the lab of Dr. Jim Carrington at Oregon State University. “Before I started working in the lab, I hadn’t thought about working with plants. I became really interested in the research they were doing in the Carrington Lab, so I decided to go to graduate school and work in the lab as a PhD student,” explains Noah.
At the same time, Noah began pursuing a career in plant science, a new technology was emerging in the scientific community: high-throughput DNA sequencing. “We went from sequencing a few hundred DNA molecules at a time to doing millions at a time.” A year into grad school, the lab was collecting so much data that he began learning how to program and do data analysis with a computer. “I shifted pretty hard away from lab work at that point.” He hasn’t looked back since.
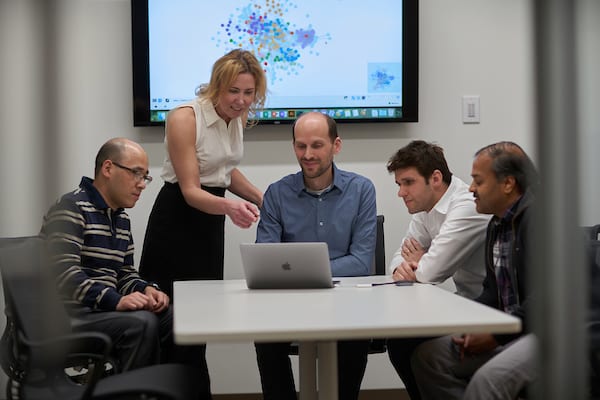
Data Science with a Mission
Today, Noah leads the Data Science Facility. His team builds computational tools that help other scientists solve big data problems. These custom tools could be anything from an algorithm, to a program, to the infrastructure that houses a particular suite of software tools. “A lot of times in science, you can’t just ask a question and use a tool that comes out of the box,” says Noah. As a result, he has made it his team’s mission to be a collaborative hub at the Danforth Center that creates tools that help bridge different areas of expertise.
There is no better example of Noah’s mission-in-action than PlantCV, an open-source image analysis software package for plant phenotyping. PlantCV helps scientists get biologically meaningful data out of hundreds of thousands of images. “The software solves a big data problem because there are a lot of dimensions to the data we can derive from images. PlantCV is able to process all of that dimensionality and distill it into meaningful data that we can do something with, in a biological sense,” explains Noah.
When Noah and his collaborators developed PlantCV, it was important to them to make it accessible to everyone. “We want our tools to be usable by everybody, meaning you shouldn’t have to be a computer expert to use the tools, and they’re freely available.” That goes for all of the tools that Noah’s team develops. As a result, PlantCV is a tool that is used across the globe. Noah’s team has even made new components of PlantCV based on user feedback. By being easily accessible, the tools developed by Noah and his team are empowering scientists around the world.
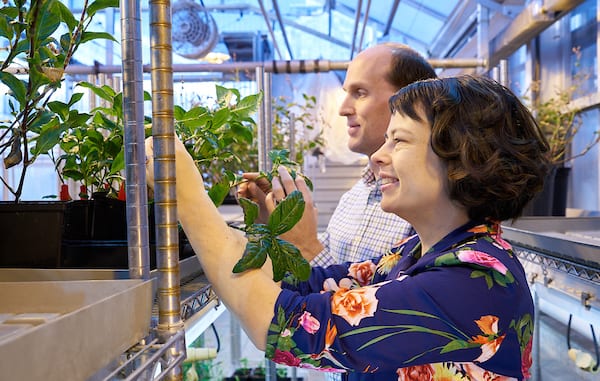
Feeding The World, Faster
“Measuring phenotypic data is a major bottleneck when it comes to crop improvement,” explains Noah. Advances in DNA sequencing technology have made it quicker and more cost efficient to measure genetic variation, but collecting phenotypic data is still expensive because it involves lots of human time. By developing imaging and image analysis technologies, Noah is making it quicker and more cost efficient for researchers to monitor phenotypes. Being able to quickly and inexpensively measure both genetic and phenotypic data together would also drastically reduce the cost and time it takes to make informed breeding decisions, ultimately helping us improve crops at a faster rate. “We envision that imaging technologies, coupled with computer vision and machine learning analysis approaches will have an impact on each step of the crop improvement process, from basic research, to breeding, to precision agriculture applications. These technologies create their own bottlenecks because the datasets are big and complex, but that’s also what makes the work so exciting because the tools and infrastructure we develop can help to tackle these issues.”